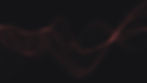
Dive Into the New Age of Accelerated Analytics
Our Services
Cloud Analytics Modernization
Cloud Analytics Modernization refers to the process of transforming traditional on-premises data analytics systems to cloud-based analytics systems. It involves migrating data, applications, and infrastructure to the cloud, utilizing cloud-based data analytics tools and platforms, and adopting modern data analytics techniques such as big data analytics, machine learning, and artificial intelligence.
The benefits of cloud analytics modernization include scalability, cost-efficiency, flexibility, and faster time-to-insight. With cloud-based analytics, organizations can easily scale their data analytics infrastructure up or down based on their needs, and pay only for the resources they use, reducing costs. Cloud analytics also offer flexibility in terms of accessing data from anywhere, anytime, and on any device, enabling remote work and collaboration.
Moreover, cloud analytics modernization enables organizations to take advantage of modern data analytics techniques such as big data analytics, machine learning, and artificial intelligence. These techniques allow organizations to analyze large volumes of data, identify patterns and insights, and make data-driven decisions in real-time.
To embark on cloud analytics modernization, organizations need to evaluate their current analytics systems and identify areas that need improvement. They should also assess the cloud-based analytics tools and platforms available in the market and choose the ones that best fit their needs. It's also essential to ensure data security and compliance while migrating data to the cloud. Overall, cloud analytics modernization can help organizations stay competitive in today's data-driven world.
Data Science Acceleration
Data Science Acceleration refers to the process of speeding up the development and deployment of data science models. The field of data science is rapidly evolving, and organizations need to be able to develop and deploy models quickly to stay competitive. Data science acceleration involves adopting tools and techniques that help organizations build and deploy models faster and more efficiently.
One key aspect of data science acceleration is the use of automation and machine learning tools. These tools can automate the data cleaning and preparation process, feature selection, and model selection, enabling data scientists to focus on higher-level tasks such as model tuning and deployment.
Another aspect of data science acceleration is the use of cloud-based data science platforms. These platforms provide a scalable and cost-effective solution for organizations that need to build and deploy models quickly. They offer a range of tools and services, such as machine learning libraries, data visualization tools, and data storage, that can help data scientists streamline the model development process.
Moreover, data science acceleration requires a collaborative approach between data scientists, data engineers, and other stakeholders. Collaboration tools such as Jupyter Notebooks and GitHub enable teams to work together on model development and deployment, reducing the time it takes to bring a model to production.
Finally, data science acceleration requires a focus on best practices and reproducibility. By using version control systems and documenting the model development process, organizations can ensure that their models are transparent, reproducible, and easy to maintain.
Overall, data science acceleration is essential for organizations that want to stay ahead in today's data-driven world. By adopting the right tools, techniques, and practices, organizations can accelerate the development and deployment of data science models, leading to better insights, faster decision-making, and improved business outcomes.
Regenerate response
Versatility in Application
Versatility in application in Data Science refers to the ability of data science techniques and tools to be applied in various fields and industries. Data science techniques and tools are versatile, which means they can be used to solve problems in a wide range of domains such as healthcare, finance, marketing, sports, social media, and many more.
In healthcare, data science can be used to analyze patient data and identify patterns, leading to better diagnoses, treatment, and outcomes. In finance, data science can be used for fraud detection, risk assessment, and investment analysis. In marketing, data science can be used to analyze customer behavior and preferences, leading to better targeting and personalization. In sports, data science can be used to analyze player performance, team strategies, and game outcomes.
The versatility of data science is due to the fact that data is being generated in every field, and organizations need to analyze this data to make informed decisions. Data science techniques such as machine learning, data mining, and statistical analysis can be applied to any data set, regardless of the domain or industry. Moreover, data science tools such as Python, R, and SQL are programming languages that can be used to work with data in any field.
The versatility of data science is also driven by the availability of cloud-based data science platforms, which provide access to data science tools and techniques on a pay-per-use basis. These platforms enable organizations to scale their data science efforts up or down based on their needs and pay only for the resources they use.
Overall, the versatility of data science is a significant advantage, as it enables organizations to extract insights and make informed decisions across a wide range of domains and industries.
Full Customer Experience Service
Full Customer Experience Service Data Science involves applying data science techniques to analyze and extract insights from a comprehensive dataset that includes all customer interactions and touchpoints with a company's products and services. It involves gathering, processing, and analyzing data from various sources such as customer feedback, social media, sales data, and customer service interactions to gain a comprehensive understanding of the customer experience.
The goal of Full Customer Experience Service Data Science is to identify trends and patterns in customer behavior and preferences, understand customer pain points, and gain insights into customer needs and expectations. With this information, organizations can make data-driven decisions to improve the customer experience, increase customer satisfaction, and drive business growth.
To conduct Full Customer Experience Service Data Science, organizations need to collect data from a variety of sources, clean and process the data, and apply appropriate data science techniques such as data mining, machine learning, and natural language processing to extract insights from the data.
Some examples of data science techniques that can be applied to Full Customer Experience Service Data Science include:
-
Text mining and sentiment analysis: This technique involves analyzing customer feedback and social media posts to extract insights on customer sentiment and opinions about a company's products and services.
-
Customer segmentation: This technique involves dividing customers into distinct groups based on their behavior, preferences, and other characteristics to gain a better understanding of their needs and expectations.
-
Predictive analytics: This technique involves using historical data to predict future customer behavior and trends, allowing organizations to make data-driven decisions about future product development and marketing strategies.
Overall, Full Customer Experience Service Data Science can help organizations gain a comprehensive understanding of the customer experience, identify opportunities for improvement, and make data-driven decisions to drive business growth and increase customer satisfaction.

We Integrate With Your Ecosystem
At The AI University Montana, LLC we are dedicated to providing students with a comprehensive education in artificial intelligence. Our cutting-edge curriculum is designed to help students develop the skills they need to succeed in the world of AI. In addition to our regular course offerings, we also offer an internship program that provides students with the opportunity to gain real-world experience under the guidance of experienced instructors. Our program offers hands-on experience in AI development, data analysis, and machine learning. We are proud to be one of the few universities to offer such an internship program.